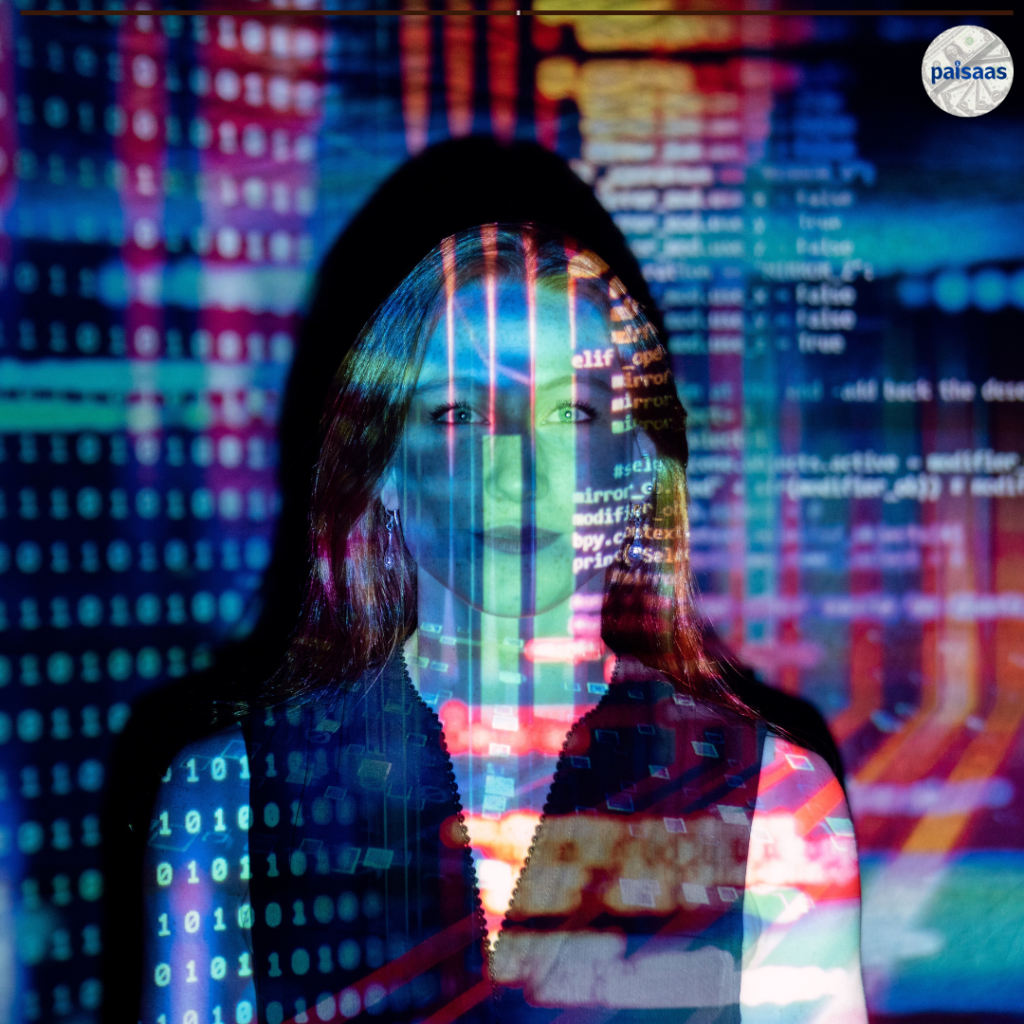
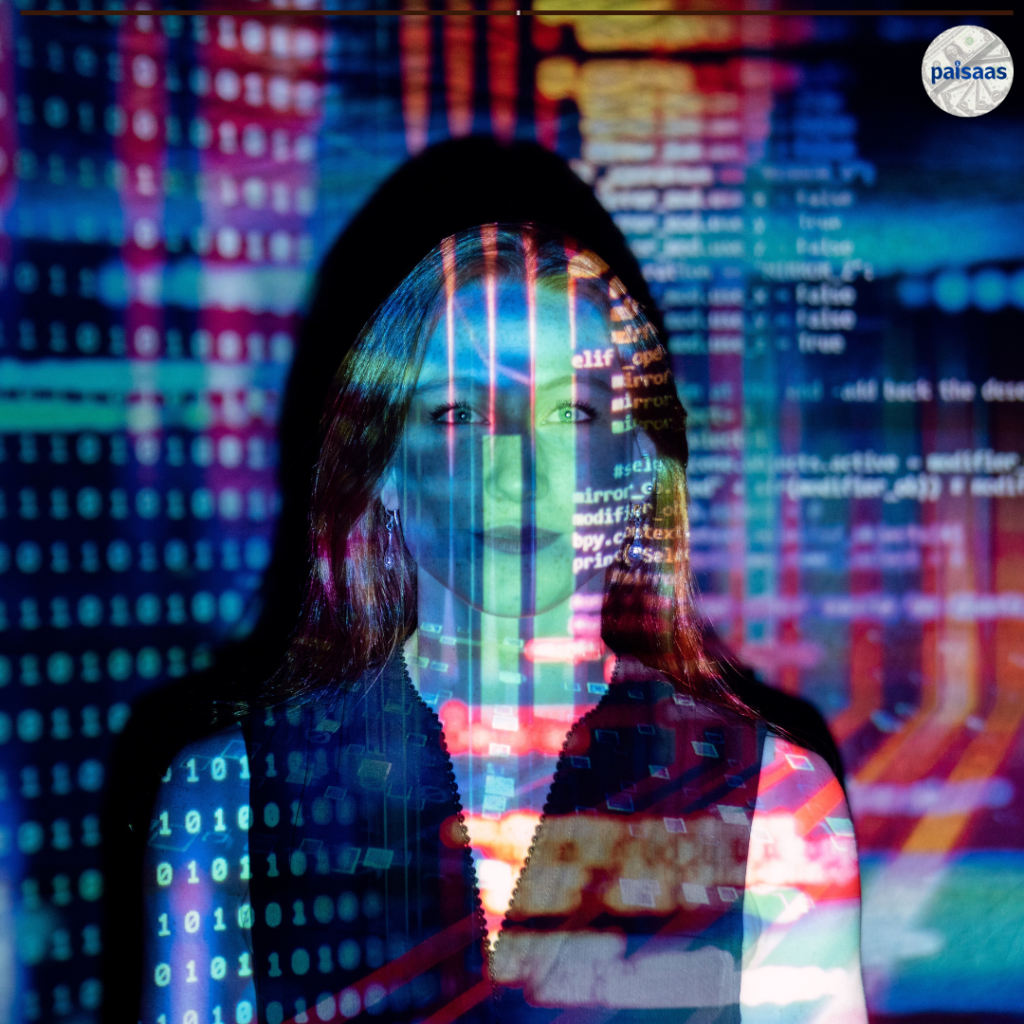
Unintended Bias in AI: Addressing Ethical Concerns and Mitigating Algorithmic Bias
Unintended Bias in AI: Addressing Ethical Concerns and Mitigating Algorithmic Bias
Introduction
As AI models continue to shape various domains, concerns regarding unintentional bias and ethical implications have gained significant traction. AI models, particularly machine learning algorithms, have the potential to propagate and amplify biases found in training data. This article delves into the mechanisms behind bias propagation in AI models and highlights essential steps to mitigate these biases, fostering the development of ethical and fair AI systems.
Unintentional Bias Propagation
Unintended bias can permeate AI models through various avenues. Firstly, biased training data can contribute to the perpetuation of discriminatory patterns. If the training data contains biased information or reflects societal prejudices, the AI model can unintentionally learn and reproduce these biases. Additionally, bias can be introduced through the algorithms themselves, such as biased feature selection, biased optimization objectives, or biased assumptions. It is crucial to acknowledge and address these mechanisms to prevent the perpetuation of bias in AI models.
Types of Bias in AI
Several types of bias can manifest in AI models. Sampling bias occurs when the training data fails to represent the entire population accurately, resulting in underrepresentation or overrepresentation of specific groups. Stereotype bias arises when AI models generalize from biased training data, leading to biased predictions or decisions. Proxy bias can also be a concern, where the use of proxies or correlated factors that themselves carry biases can result in discriminatory outcomes. Recognizing these types of bias is vital for effective mitigation strategies.
Steps to Mitigate Bias in AI : To mitigate bias in AI models, several critical steps can be taken:
- Diverse and Representative Training Data: Ensuring that training data is diverse and representative of the target population is key to mitigating bias. Incorporating data from various sources and demographics helps minimize underrepresentation or overrepresentation of specific groups.
- Bias Detection and Evaluation: Rigorous evaluation of training data and AI models is necessary to identify and quantify bias. Employing specific metrics and evaluation techniques that focus on fairness and bias aids in detecting and addressing potential issues.
- Data Preprocessing and Cleaning: Applying preprocessing techniques to remove or mitigate biased patterns in the training data can help reduce bias propagation. This may involve anonymizing sensitive attributes and using techniques like data augmentation to balance representation.
- Algorithmic Fairness Techniques: Utilizing fairness-aware algorithms and techniques can assist in reducing bias in AI models. Adjusting optimization objectives, reweighting training examples, or incorporating fairness constraints during the learning process can help address bias.
- Regular Monitoring and Auditing: Continuous monitoring and auditing of AI systems are crucial to identify and address any emerging biases post-deployment. Regular assessments help ensure ongoing fairness and ethical considerations in AI decision-making.
Transparent and Explainable AI : Ensuring transparency and explainability in AI models aids in addressing bias. Interpretable models, such as decision trees or rule-based systems, provide insights into the decision-making process, enabling better identification of biases. Employing techniques like feature importance analysis and model-agnostic interpretability methods can help uncover biases and provide explanations for AI predictions or decisions.
Ethical Responsibility and Human Oversight : Ethical guidelines and regulations play a vital role in promoting responsible AI development and deployment. Governments, organizations, and industry bodies should establish and adhere to these guidelines, setting standards for fair and unbiased AI systems. Additionally, building diverse and inclusive AI teams fosters a broader range of perspectives and experiences, facilitating the identification and mitigation of biases during AI development and evaluation stages.
Conclusion
Addressing unintended bias in AI models is crucial for developing ethical and fair AI systems. By recognizing the mechanisms behind bias propagation and implementing steps such as diverse training data, bias detection, data preprocessing, fairness techniques, transparent AI, and ethical responsibility, we can move towards creating AI systems that promote fairness and mitigate unintended biases.