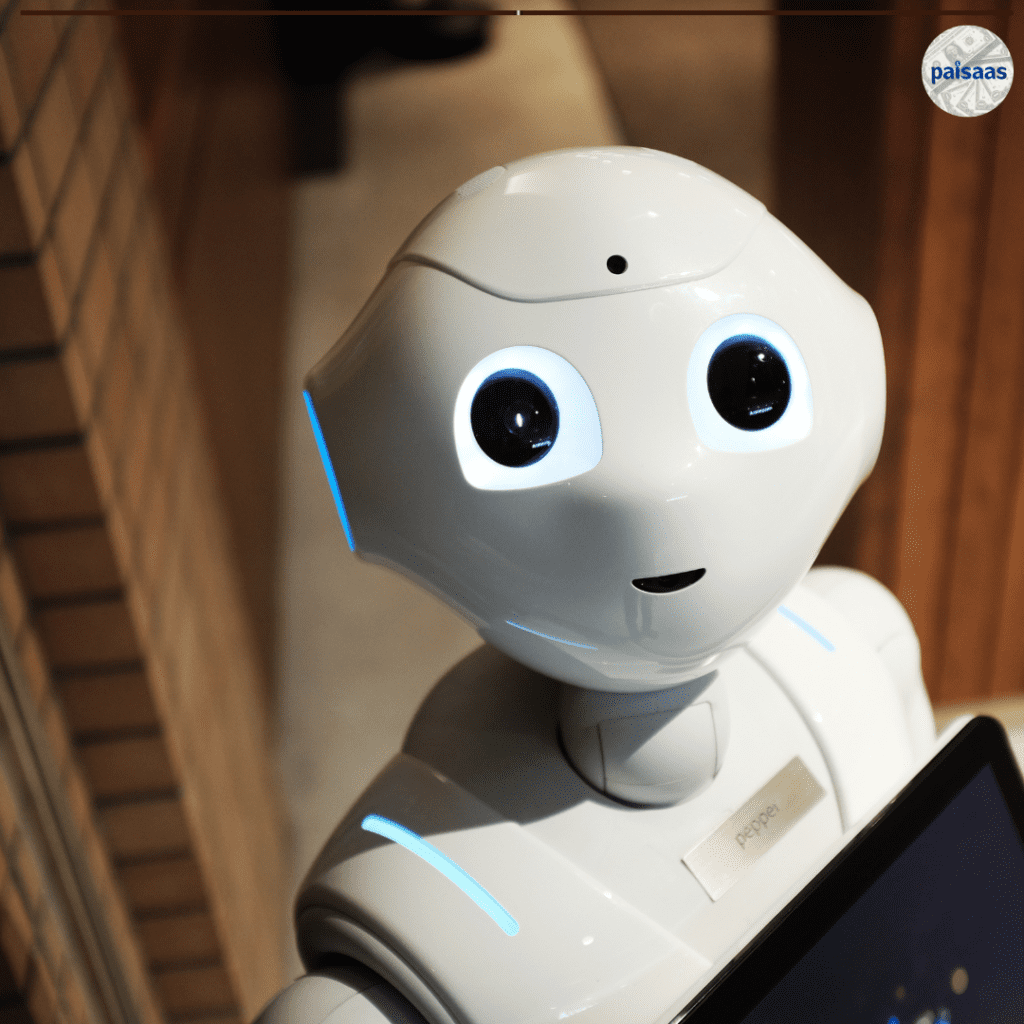
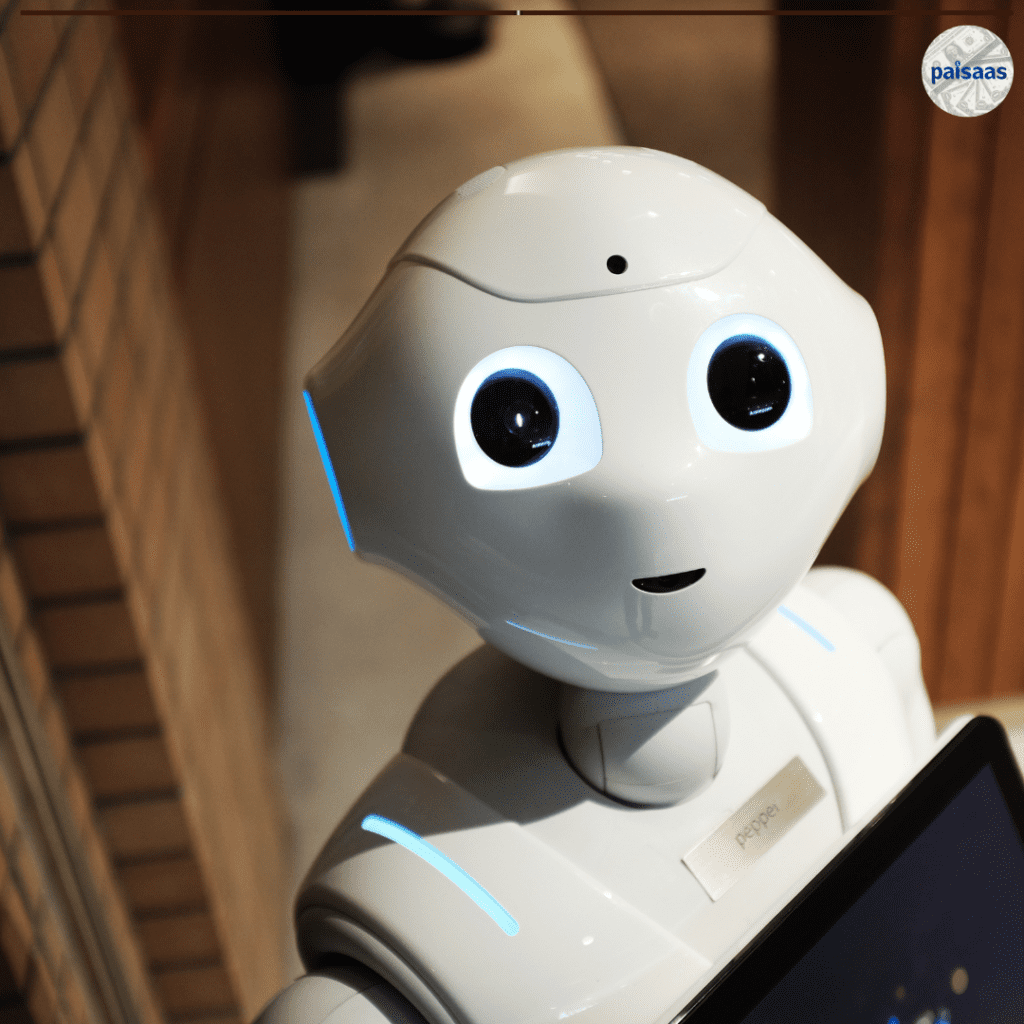
Explaining the Importance of Explainable AI in High-Stakes Applications
Explaining the Importance of Explainable AI in High-Stakes Applications
Introduction
As artificial intelligence (AI) systems continue to advance, understanding their decision-making processes becomes increasingly crucial, especially in high-stakes fields like healthcare and autonomous vehicles. Explainable AI (XAI) seeks to demystify AI algorithms, providing transparency and ensuring accountability and trust in critical applications. In this article, we will delve into the significance of explainable AI in high-stakes domains, explore its ethical considerations, discuss techniques for achieving explainability, and highlight the benefits it brings to various applications.
The Need for Explainable AI
In high-stakes applications, the demand for explainability in AI systems becomes paramount. In the field of healthcare, AI algorithms play a significant role in assisting with diagnoses, treatment plans, and patient outcomes. Understanding the rationale behind these AI-driven decisions is crucial for healthcare professionals and patients alike. Explainable AI helps build trust, allowing clinicians to validate and improve the accuracy of AI-generated insights while enabling patients to comprehend the reasoning behind their medical recommendations.
Similarly, in the realm of autonomous vehicles, where AI systems make split-second decisions that impact the safety of passengers, pedestrians, and other vehicles on the road, explainability becomes essential. Drivers, regulatory bodies, and the general public need to understand the decision-making process of AI systems to ensure transparency, identify potential biases, and address safety concerns.
Ethical Considerations
Explainable AI also plays a significant role in addressing ethical considerations associated with AI algorithms. One such concern is the presence of biases within AI systems. If AI algorithms are trained on biased data, they may inadvertently perpetuate and amplify societal biases, leading to discriminatory outcomes. By providing explanations, XAI allows for the identification and rectification of biases, promoting fairness and equity.
Moreover, legal and regulatory compliance is a critical aspect, particularly in industries such as finance and healthcare, which are subject to strict regulations. Explainable AI enables organizations to demonstrate transparency and accountability in their AI-driven decision-making processes, ensuring adherence to ethical and legal standards.
Techniques for Achieving Explainable AI
Several techniques contribute to achieving explainability in AI systems. One approach involves utilizing inherently interpretable models, such as decision trees or rule-based systems, which provide clear explanations of their outputs. These models operate on explicit rules that can be easily understood by humans.
Another technique is post-hoc interpretability, which involves applying methods to interpret the decisions made by complex AI models. Techniques such as feature importance analysis and visualization methods help humans understand the factors influencing AI decisions.
Rule extraction is another strategy for achieving explainability. It involves extracting decision rules from AI models, allowing humans to comprehend how inputs are translated into outputs. This approach enhances transparency and provides insights into the decision-making process.
Benefits in High-Stakes Applications
Explainable AI offers numerous benefits in high-stakes applications. In healthcare, it promotes trust between healthcare professionals and AI algorithms. Doctors can validate AI-generated insights, improving patient care and outcomes. Patients, on the other hand, gain transparency and understanding, knowing why specific treatment plans or diagnoses are recommended.
For autonomous vehicles, explainable AI plays a pivotal role in building trust and acceptance. By understanding the decision-making process of AI systems, drivers, pedestrians, and regulatory bodies can assess the reliability and safety of self-driving cars, fostering public confidence and paving the way for widespread adoption.
Additionally, explainable AI is critical for addressing safety concerns and avoiding catastrophic failures. In high-stakes environments such as power grids or nuclear facilities, understanding the decision-making process of AI systems is vital to prevent accidents and ensure public safety.
Conclusion
Explainable AI holds immense importance in high-stakes applications, providing transparency, trust, and accountability. By understanding AI algorithms’ decision-making processes, we can address biases, comply with regulations, and foster ethical implementation, ensuring the responsible use of AI technologies in critical domains. Embracing explainable AI enables us to harness the full potential of AI while mitigating risks and ensuring the well-being of individuals and society as a whole.